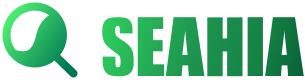
AI-driven Carbon Footprint Tracking operates on three core principles:
Data Fusion: Algorithms integrate information from thousands of sources, including energy bills, credit card transactions, and IoT sensors in homes and vehicles . For example, smart thermostats like Nest or smart plugs can track electricity usage, while apps like Tred analyze spending patterns to estimate emissions from purchases .
Machine Learning: Models learn from historical data to identify hidden patterns. Google’s DeepMind, for instance, developed tools that predict emissions from transportation and energy use by analyzing GPS data and weather forecasts. These systems improve accuracy over time, adjusting for variables like regional energy grids or driving habits.
Real-Time Adaptation: Unlike static calculators, AI updates carbon estimates hourly. If you switch to an electric vehicle or buy locally sourced groceries, the system instantly reflects these changes in your footprint .
This technology isn’t just for individuals. The U.S. Environmental Protection Agency (EPA) uses AI to analyze nationwide emissions data from industries and power plants, feeding into tools that ordinary citizens can access . By merging personal data with national trends, AI creates a holistic view of environmental impact.
For everyday Americans, Carbon Footprint Tracking via AI translates to actionable insights:
Effortless Monitoring: Apps like EcoTrack connect to bank accounts and credit cards to automatically categorize purchases. A $5 coffee might show up as 0.3 kg CO₂e (carbon dioxide equivalent) due to transportation and packaging .
Behavioral Feedback: AI identifies habits that contribute to high emissions. If your commute emits 10 kg CO₂e daily, the system might suggest carpooling or public transit routes with lower impact .
Climate Literacy: Users learn how small changes ripple into larger impacts. For example, replacing a gas stove with an induction cooktop reduces emissions by 20%—a fact AI might highlight during energy bill analysis .
The benefits extend beyond individuals. Businesses use AI to optimize supply chains, reducing emissions from manufacturing and shipping. When you order a product online, AI might reroute it through a greener logistics network without notifying you .
Despite its promise, Carbon Footprint Tracking via AI faces hurdles:
Data Privacy: Collecting personal information raises concerns. Companies like Wiliot address this by anonymizing data and allowing users to opt out of tracking .
Model Accuracy: AI struggles with fragmented data sources. For instance, emissions from international flights or imported goods rely on estimates, not real-time measurements .
Equity Gaps: Low-income households may lack access to smart devices or high-speed internet, limiting AI’s reach .
To overcome these issues, organizations like the EPA partner with tech companies to standardize data protocols and improve transparency . Meanwhile, open-source tools like Carbon Tracker enable developers to build eco-friendly AI models .
The future of Carbon Footprint Tracking is already here. By 2027, NOAA plans to integrate AI into its climate models, offering hyper-local emissions forecasts—like predicting how a neighborhood’s energy use will impact regional carbon levels . Companies like Meteomatics are testing AI systems that analyze satellite imagery to track deforestation and agricultural emissions in real time .
For ordinary Americans, this means more power to make informed choices. Imagine a future where your phone alerts you that charging your EV during off-peak hours cuts emissions by 40%, or a grocery app highlights products with the lowest lifecycle carbon scores . AI isn’t just tracking footprints—it’s turning passive data into active climate solutions.